The accurate delineation of land boundaries is a critical step in identifying land-use and planning their management. Particularly for croplands, this delineation enables farmers and agribusinesses to better estimate the land area for the efficient use of agri-inputs, such as seeds, pesticides, fertilisers, and other resources and to optimise production and post-production activities.
Manual processes of land boundary identification have proved to be time consuming and labour intensive. However, advanced technology in agriculture, like remote-sensing imagery, provides in-depth and spatially explicit information on agricultural land use in real-time that would otherwise be difficult to obtain.
Satellite imaging, machine learning, and AI in agriculture are equipping actors in the agroecosystem with a historical record of the distribution of cultivated crops across regions. Government entities can leverage this intelligence to adequately plan the import and export of food products. Financial institutions can utilise this alternate agri-data to determine the crop a farmer is cultivating, its health, and the performance of the plot to appraise loan proposals. Agribusinesses can remotely monitor and manage their farms at every stage of crop production without having to visit the fields frequently, especially in the light of the current predicament caused by the pandemic.
What is the Use of Land Boundary?
To obtain accurate data for any of these assessments and arrive at conclusive insights, the exact boundaries of the farm plot plays a crucial role. In recent years, satellite imaging data, specifically such as those from Landsat-8 (optical), Sentinel-1 (RADAR), and Sentinel-2 (optical) are used to identify the location, size, and spatial extent of the farm land. This data is then combined with weather information, to improve crop type classification and monitor them in real time.
Advanced technology in agriculture that also integrates other information, such as crop type, soil type, and water stress in the region, become vital to devising an effective farming plan and to develop and monitor agricultural policies and schemes for farmers. They empower producers with other capabilities, such as yield prediction, crop stage forecasting, crop health estimation, identification of planting and harvesting dates, water stress estimation, soil moisture estimation, and irrigation scheduling.
While crop land boundaries are easier to delineate and digitise for large landholdings, it becomes increasingly difficult for small landholdings, particularly in certain emerging countries and regions. The absence of well-defined boundaries makes other tasks, such as land-use classification, digitisation of land titles, or crop classification more cumbersome and error prone.
Land boundaries, hence, are the bedrock for deriving critical land cover information, particularly for agriculture. Data scientists utilise Earth observation data to detect land boundaries, which further enable them to classify and evaluate land use/land cover (LULC) patterns to detect vegetation from other forms of land cover. Going a step further, the satellite data and applications of advanced technology in agriculture like machine learning also allow them to identify and classify crops. It further empowers producers to remotely monitor the health, stress, and yield of the crop periodically and ensure high productivity throughout the crop cycle.
Why Is Land Boundary Detection Required?
The manual process of demarcating plot boundaries is prone to human errors and requires in-depth annotation skills. Once the plots have been delineated, they also need to be updated periodically to monitor the changes in real-time.
Automatic detection of land boundaries facilitates the digitisation of all individual plots across the country with minimal human correction. This would be particularly beneficial in countries like India, where digital records of land boundaries are not extensively available. Although some states have taken the initiative to digitise the records at a survey number level, the survey numbers often comprise several smaller land parcels, the boundaries for which are manually and informally demarcated.
The added advantage of this digital process is that all the information regarding a particular farm can be consolidated based on the plots detected by the land boundary detection model. The exact delineation of plots helps in obtaining more precise information concerning the crop type or yield estimate. Besides, this algorithm will help in detecting the change in the area of a farmer’s plot over the years. In the event of an earthquake, drought, flooding, or other natural disasters, the algorithm can help the concerned parties to detect the damage to the plot or the crops. Likewise, corporate enterprises and builders undertaking governance and surveillance will benefit to a significant extent with respect to area planning and management and land value assessment.
Current Limitations of Detecting Land Boundaries
Advanced technology in agriculture has evolved tremendously in recent decades and have also offered scope for further advancements. Nonetheless, remote sensing images have their own limitations. They usually have a very low image resolution, level of noise, and a massive volume that takes up considerable digital storage space. The properties of these images drastically change from one region to another region depending on the land area’s properties.
Land boundary detection for landholdings that are disintegrated and nonuniform is, in fact, an arduous task as their boundaries do not have a defined shape or size, particularly in countries like India where landholdings are small in size and dense as well. Image processing techniques like segmentation and edge detection, which works well on general images, may not yield accurate results for remote sensing images. Two adjacent plots of vegetation may hold good potential for us to find the land boundaries based on the difference of color and texture, but these properties may not be prominent always when both plots have the same crop. This concern also applies to those plots with multiple crops as well. Due to these factors, land boundary detection using remote sensing images is still an open research problem. There is no such robust solution which works perfectly across the different geographical regions at the present.
CropIn’s Deep-Learning Engine for Detecting Land Boundaries
CropIn has developed a proprietary state-of-the-art land boundary detection algorithm by combining deep learning and with classical image processing techniques. The algorithm primarily consists of three modules: a) boundary line extraction algorithm, b) post processing algorithm, and c) polygonization for farm plot extraction. The deep learning model is trained using Google satellite images as inputs, which are RGB raster images with a spatial resolution as high as 0.5 m in some places (depending on data sources), along with annotated land boundaries as labels.
CropIn has deployed this deep-learning model for boundary detection in the Indian state of Maharashtra. We procured geo-referenced land record maps of villages in the state from Maharashtra Remote Sensing Application Centre (MRSAC), which provides us with manually-defined land boundaries for the different survey numbers. These land records serve as the first layer of ground truth and the baseline to build and train the deep-learning models. We then utilise Google Earth Engine’s high-resolution satellite imagery to refine the boundaries through the process mentioned above.
We scaled our algorithm by introducing parallel processing built on AWS Batch, which spins up parallel virtual machines to do the prediction on a large area simultaneously. A ‘r5.xlarge’ AWS EC2 Spot instance was used to do the prediction. Currently, our system can detect the land boundary for nearly 300,000 sq kms of area in 6-7 hours. A sample of the land boundaries detected by our proprietary algorithm is shown in the figure below.
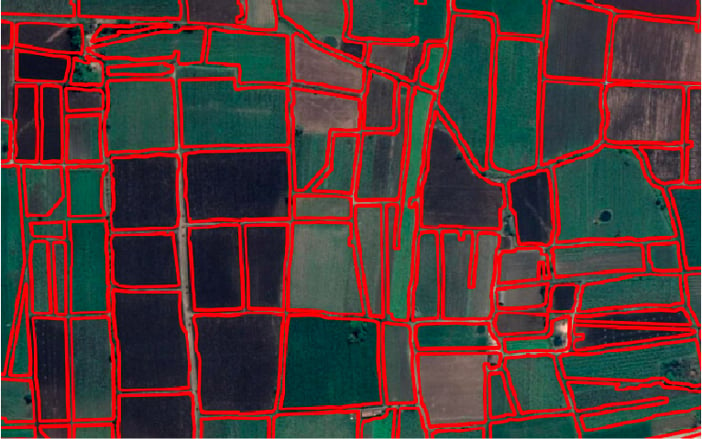
Who can leverage these Land Boundaries?
It empowers government bodies, financial institutions, and other stakeholders to arrive at data-driven decisions based on timely and more accurate information, which contributes to increased productivity, efficiency, and profitability.